


Where can you use drones in Rust? Everything to Know About Drones in Rust
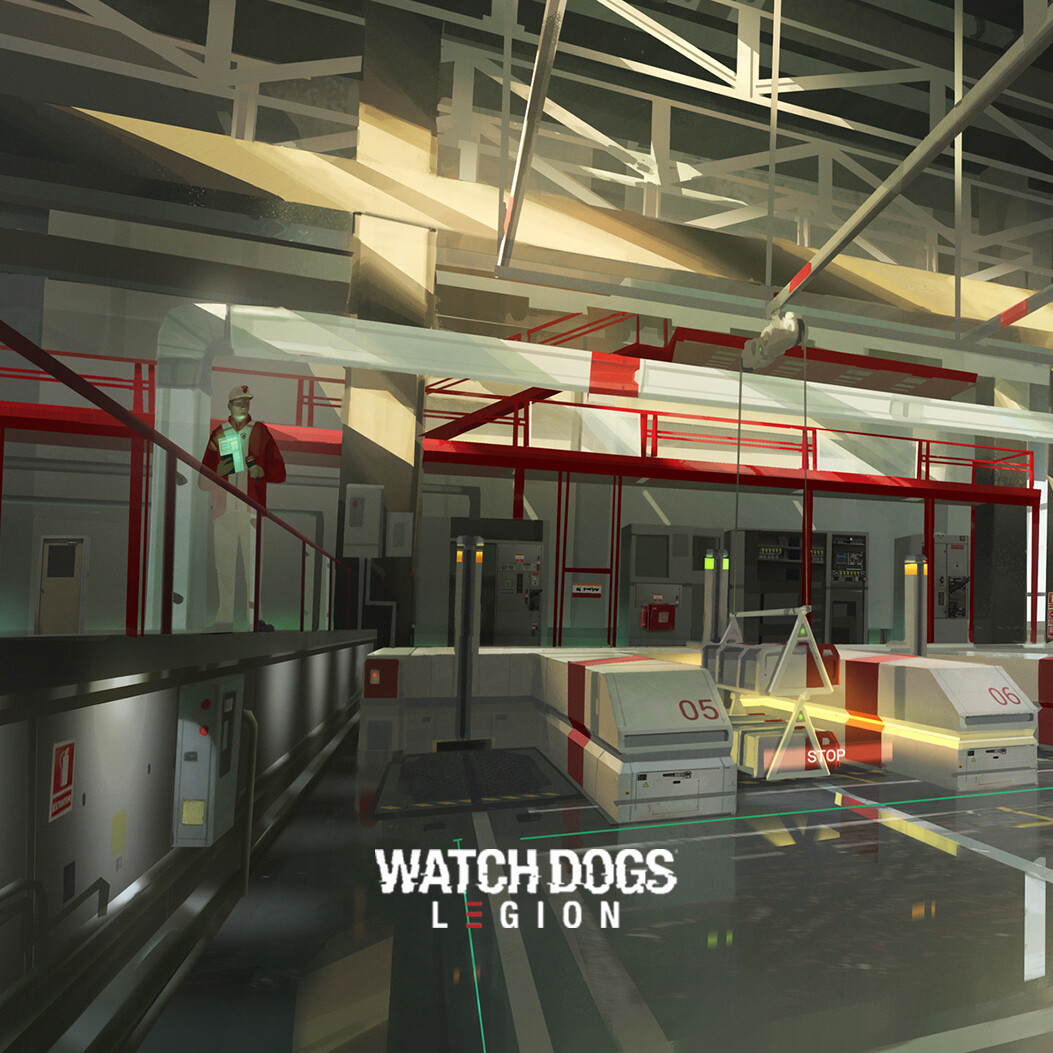
Click on them to see what items they sell, which will be shown in the bottom right corner of the screen, then make a purchase whenever the desired product is found. The research team included Bushra Jalil, Giuseppe Riccardo Leone, Massimo Martinelli, Davide Moroni, Maria Antonietta Pascali and Andrea Berton with support from Istituto di Scienza e Tecnologie dell’Informazione “Alessandro Faedo” and the Istituto di Fisiologia Clinica CNR, in Pisa, Italy.When activating one of the control panels, it will bring up a map showing all the vending machines that the drone can access. The results showed that the use of multi-modal imaging and of convolutional networks may improve the efficiency of drone surveying of the electrical infrastructure, and may open the way to the development of a novel monitoring payload (to be embedded in UAV) able to provide accurate and fast detection of faults and anomalies in power lines and insulators. With an average of 0.98 similarity index, and 0.95 Dice similarity coefficient, in different background conditions, the method results proved effective for the detection of power lines.
#Rust drone station manual#
The team published a paper describing the different approaches used to detect and classify insulators, and to analyse power transmission lines with details about the experimentation and the results achieved.ĭiscussion and Conclusions: Results from the study were computed with different statistical parameters by comparing the performance of obtained output with the manual ground truth. For insulators, researchers used recursive CNN (Convolutional Neural Networks) to detect insulators in visible images and to classify their status. To inspect temperature anomalies, the team performed multi-modal imaging integrating IR and visible data to identify faults and anomalies in power equipment. Infrared imaging, which is invariant to large scale and illumination changes in the real operating environment, supported the identification of faults in power transmission lines while a neural network is adapted and trained to detect and classify insulators from an optical video stream. State-of-the-art computer vision methods were used to highlight expected faults (i.e., hot spots) or damaged components of the electrical infrastructure (i.e., damaged insulators). Methods were developed to classify insulators from a drone’s optical video stream and to detect hot spots along with the power lines from visible and infrared images. They utilized visible images to extract power lines and rusted insulators, and IR images relayed by the drone to identify hot spots in the processing pipeline. In this work the researchers carried out the detection of hot spots and rusted insulators from sequences of images acquired by a drone surveying the electrical infrastructure. The researchers presented, algorithms and methods developed for improving the capabilities of UAV-based inspection systems with primary focus on temperature anomalies along cables, and on insulators hanging from electric towers. After detecting power lines from visible image, histogram based thresholding on the registered IR image gives expected faults. (a) Color map of IR image with semi-cluttered background, (b) Obtained hot spot in the power lines.
